Predictive Analytics in Healthcare: Transforming the Future of Medicine
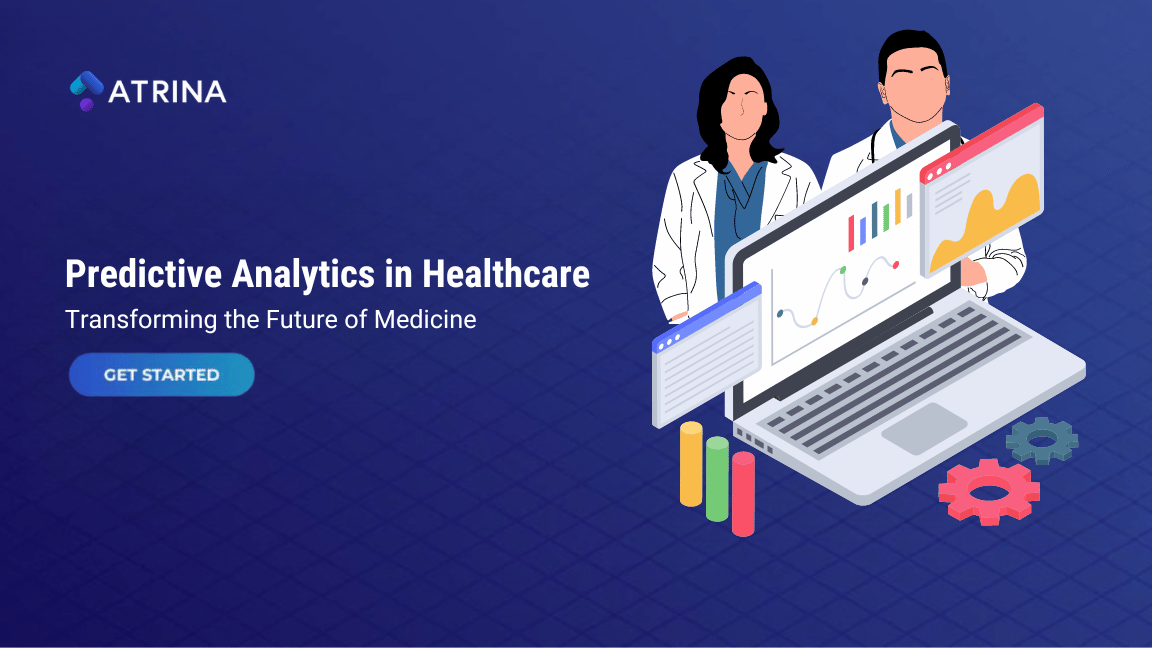
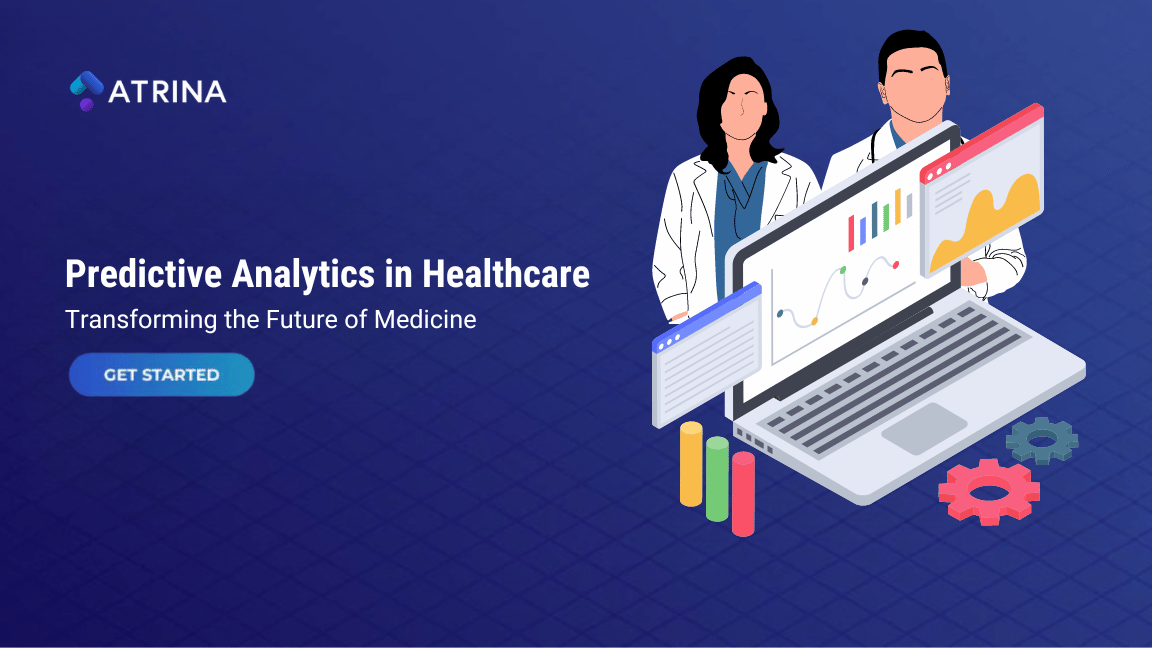
Predictive analytics in healthcare is transforming the way we approach medical treatment, disease prevention, and patient management. Leveraging vast amounts of data, predictive models can forecast health outcomes, identify at-risk populations, and personalize treatment plans, leading to more efficient and effective healthcare delivery. This blog delves into the significance, applications, and impact of predictive analytics in healthcare, backed by compelling statistics that underscore its growing influence.
Understanding Predictive Analytics in Healthcare
Predictive analytics involves the use of statistical techniques, machine learning algorithms, and data mining to analyze historical and real-time data, identify patterns, and predict future outcomes. In healthcare, this means attaching data from electronic health records (EHRs), wearable devices, genomics, and more to anticipate health events, optimize resource allocation, and improve patient outcomes.
As the healthcare industry increasingly shifts toward value-based care, predictive analytics has become a cornerstone in achieving better health outcomes at lower costs. According to a report by Grand View Research, the global predictive analytics market in healthcare was valued at USD 1.48 billion in 2020 and is expected to grow at a compound annual growth rate (CAGR) of 29.4% from 2021 to 2028, reaching USD 14.95 billion by 2028 .
Key Applications of Predictive Analytics in Healthcare
- Early Disease Detection and Prevention
- Predictive analytics plays a crucial role in identifying individuals at risk for chronic diseases like diabetes, heart disease, and cancer. By analyzing patterns in patient data, healthcare providers can intervene early, potentially preventing the onset of these conditions.
- A study published in the Journal of the American Medical Informatics Association found that predictive analytics reduced hospital readmissions by 25% by identifying high-risk patients and implementing targeted interventions.
- Personalized Treatment Plans
- Predictive models can help custom treatments to individual patients based on their unique genetic makeup, lifestyle, and other factors. This approach, known as precision medicine, enhances the effectiveness of treatments and reduces adverse effects.
- The American Society of Clinical Oncology (ASCO) reported that predictive analytics in oncology could reduce treatment costs by 20-30% while improving patient outcomes through personalized therapy regimens.
- Improving Hospital Operations
- Hospitals are increasingly using predictive analytics to optimize resource allocation, reduce patient wait times, and manage staff scheduling. For example, by predicting patient admission rates, hospitals can better manage bed availability and reduce overcrowding.
- A survey by the Society of Actuaries revealed that 47% of healthcare organizations have already adopted predictive analytics to enhance operational efficiency, with 93% of adopters reporting a positive impact.
- Reducing Readmission Rates
- Readmissions are costly for healthcare providers, and predictive analytics can help reduce these rates by identifying patients at high risk of being readmitted. For instance, predictive models can analyze patient demographics, medical history, and post-discharge care plans to determine which patients are most likely to require readmission.
- According to a study by Healthcare Financial Management Association (HFMA), hospitals using predictive analytics to manage readmissions saw a 10-20% reduction in readmission rates, saving millions of dollars annually.
- Enhancing Population Health Management
- Population health management focuses on improving the health outcomes of specific patient groups, such as those with chronic diseases or those in vulnerable populations. Predictive analytics can identify trends and risk factors within these groups, enabling targeted interventions and improved health outcomes.
- The Centers for Disease Control and Prevention (CDC) reported that predictive analytics could reduce the incidence of chronic diseases by up to 15% through early intervention and targeted care strategies.
- Combatting the COVID-19 Pandemic
- Predictive analytics has been instrumental in managing the COVID-19 pandemic. From predicting infection hotspots to optimizing vaccine distribution, predictive models have played a vital role in controlling the spread of the virus.
- A report by McKinsey & Company highlighted those predictive analytics enabled healthcare systems to manage the surge in COVID-19 cases more effectively, reducing the strain on resources by up to 20%.
Challenges in Implementing Predictive Analytics in Healthcare
Despite its potential, the adoption of predictive analytics in healthcare faces several challenges:
- Data Quality and Integration
- Healthcare data is often fragmented across different systems, making it difficult to integrate and analyze comprehensively. Ensuring data quality, consistency, and completeness is crucial for accurate predictions.
- Privacy and Security Concerns
- The use of patient data in predictive analytics raises concerns about privacy and data security. Healthcare organizations must adhere to stringent regulations, such as HIPAA in the United States, to protect patient information while leveraging predictive models.
- Interpretability of Predictive Models
- Predictive models, especially those based on machine learning, can be complex and difficult to interpret. Healthcare providers need to understand how these models make predictions to trust and effectively use them in clinical decision-making.
- Cost and Resource Allocation
- Implementing predictive analytics requires significant investment in technology, infrastructure, and skilled personnel. Smaller healthcare organizations may struggle to justify these costs, particularly if they lack the resources to maintain and update predictive models.
The Future of Predictive Analytics in Healthcare
As technology continues to evolve, the future of predictive analytics in healthcare looks promising. Here are some trends to watch:
- Integration of AI and Machine Learning
- The integration of artificial intelligence (AI) and machine learning with predictive analytics will enable even more accurate and personalized predictions. For example, AI-driven models can analyze vast amounts of unstructured data, such as medical images and clinical notes, to uncover insights that were previously inaccessible.
- Expansion of Wearable Devices
- Wearable devices, such as smartwatches and fitness trackers, are generating an increasing amount of real-time health data. This data, when integrated with predictive analytics, can provide continuous monitoring and early warnings of potential health issues, empowering patients to take proactive steps in managing their health.
- Genomic Data Integration
- The incorporation of genomic data into predictive models will enhance the precision of personalized medicine. By analyzing genetic information alongside clinical data, healthcare providers can predict an individual’s risk for specific diseases and tailor preventive measures accordingly.
- Collaborative Health Ecosystems
- Predictive analytics will drive the development of collaborative health ecosystems, where multiple stakeholders, including healthcare providers, payers, and technology companies, work together to improve patient outcomes. These ecosystems will facilitate the sharing of data and resources, leading to more comprehensive and effective healthcare solutions.
Conclusion
Predictive analytics is dignified to transform healthcare by enabling more proactive, personalized, and efficient care. From early disease detection to improving hospital operations, predictive models are helping healthcare providers make better decisions, reduce costs, and enhance patient outcomes. However, the successful implementation of predictive analytics requires overcoming challenges related to data integration, privacy, and model interpretability. As the healthcare industry continues to use predictive analytics, it is essential to balance the potential benefits with the ethical considerations and practical challenges that accompany this powerful technology.
The future of healthcare lies in the ability to anticipate and respond to patient needs before they arise, and predictive analytics is the key to unlocking this potential. By continuing to innovate and refine predictive models, healthcare providers can deliver higher-quality care, improve population health, and ultimately save lives.