AI-Powered Credit Scoring: Digital Evolution Of Financial Risk Management
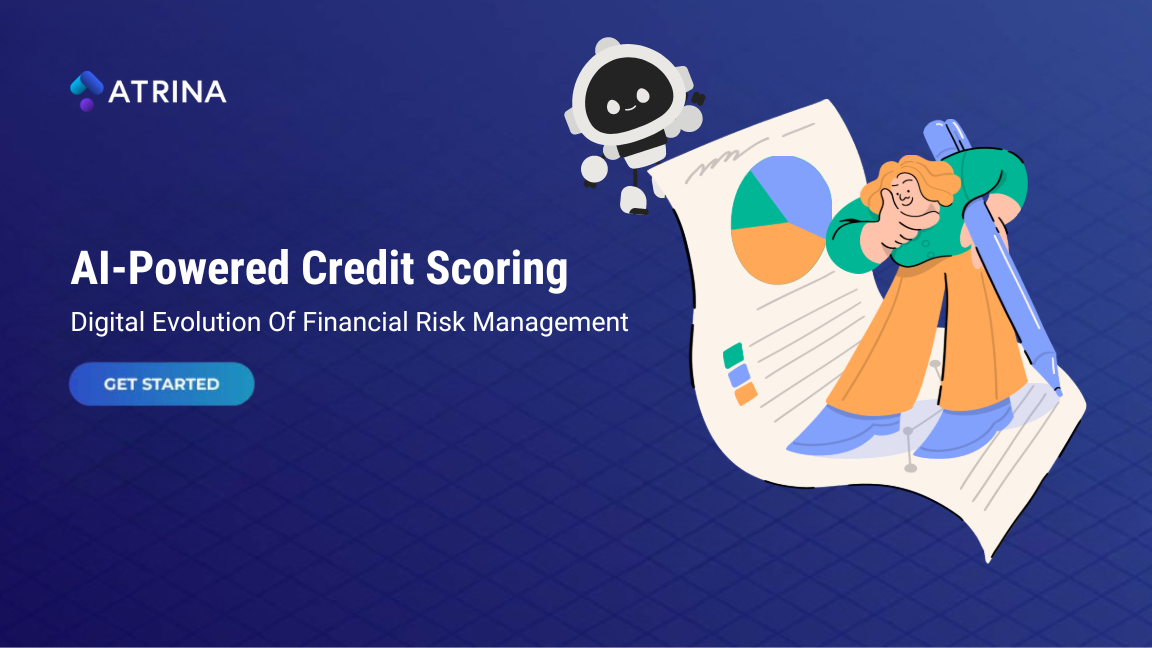
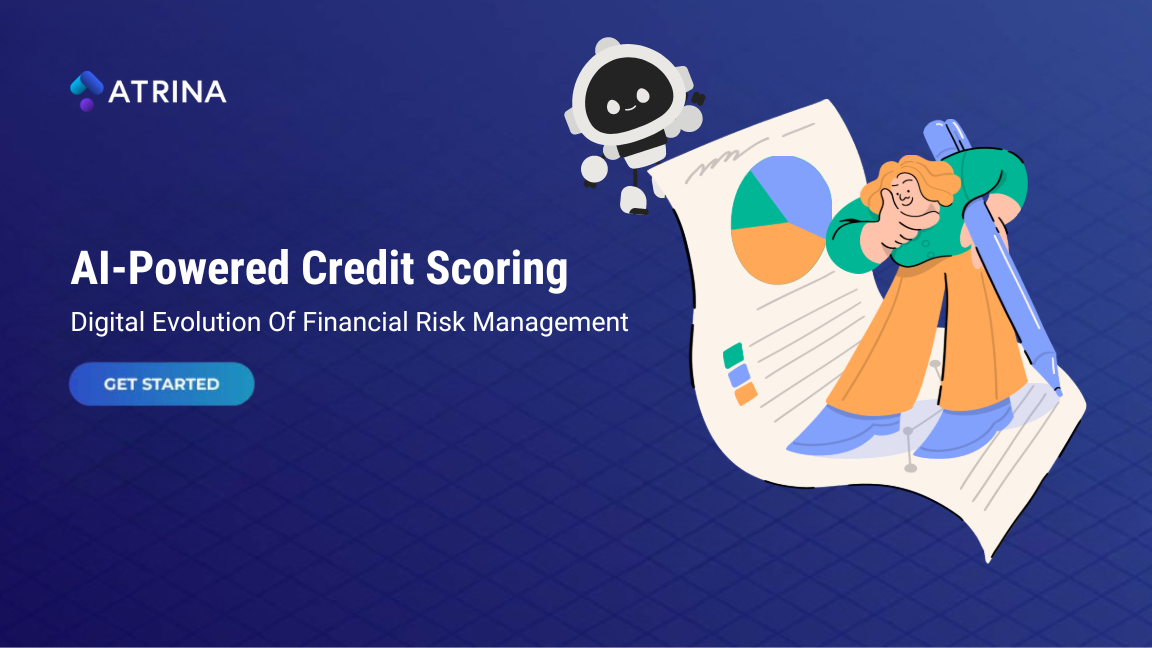
The financial sector is seeing a notable metamorphosis fueled by the swift integration of artificial intelligence (AI) in risk assessment and credit evaluation. As the global market for credit scoring services continues to expand, the integration of AI technologies has become a transformative factor. In 2022, the global market for credit scoring services was projected to reach USD 18.97 billion, with an impressive compound annual growth rate (CAGR) of 24.06% from 2023 to 2030. This statistic underscores the growing reliance on advanced credit scoring technologies, particularly AI-driven solutions, as financial institutions and fintech companies seek to enhance their risk assessment capabilities and improve financial inclusion.
The importance of AI in credit scoring is further highlighted by the increasing number of financial institutions and fintech companies leveraging machine learning algorithms to modernize their credit risk assessment processes. For instance, American Express, a leader in the financial services industry, has employed machine learning algorithms to analyze customer data, resulting in a 10% reduction in default rates and a 15% increase in the number of customers approved for credit. Similarly, innovative lending platforms like Upstart have incorporated non-traditional data points, such as education and employment history, to assess creditworthiness, leading to a significant reduction in default rates compared to traditional credit scoring methods.
This blog highlights the role of AI in credit scoring, exploring its impact on financial risk management, the benefits it offers, the challenges it presents, and its potential to reform the future of the financial industry.
The Evolution of Credit Scoring: From Traditional Methods to AI-Driven Solutions
Credit scoring has long been a critical component of financial risk management, enabling lenders to assess the creditworthiness of individuals and businesses. Traditionally, credit scoring models relied heavily on a limited set of data points, such as credit history, income, and existing debts, to determine an individual’s or business’s credit score. These traditional models, while effective to some extent, have been criticized for their reliance on historical data and their inability to consider a broader range of factors that might influence creditworthiness.
The limitations of traditional credit scoring methods have become increasingly apparent in fintech domain, where consumers and businesses alike are engaging in more complex and diverse financial activities. This has led to the need for more sophisticated and accurate credit scoring models that can take into account a wider array of data points and provide a more comprehensive assessment of credit risk.
This is where AI-driven credit scoring solutions come into play. By leveraging machine learning algorithms and big data analytics, AI-powered credit scoring models can analyze vast amounts of data from various sources, including non-traditional data points such as social media activity, online behavior, and even education and employment history. This enables these models to provide a more accurate and nuanced assessment of creditworthiness, ultimately leading to better lending decisions and reduced risk for financial institutions.
Key Players in AI-Driven Credit Scoring
Several financial institutions and fintech companies have already begun to use AI in their credit scoring processes, with impressive results. Below are some real-life examples of how AI is being used to transform credit scoring:
- American Express: American Express has successfully integrated machine learning algorithms into its credit risk assessment process. By analyzing customer data, the company has been able to achieve a 10% reduction in default rates and a 15% increase in the number of customers approved for credit. This shows the effectiveness of AI in improving the accuracy of credit risk assessments and enhancing the overall lending process.
- Upstart: Upstart is a pioneering lending platform that leverages AI to assess creditworthiness by incorporating non-traditional data points such as education and employment history. This innovative approach has resulted in a significant reduction in default rates compared to traditional credit scoring methods. Upstart’s success highlights the potential of AI to improve financial inclusion by enabling lenders to consider a broader range of factors when assessing credit risk.
- ZestFinance: ZestFinance is a fintech company that focuses on using machine learning to analyze alternative data for credit scoring. By considering factors beyond traditional credit scores, such as transaction data and behavioral patterns, ZestFinance’s AI models can provide credit to individuals who might otherwise be overlooked by conventional scoring methods. This approach not only promotes financial inclusion but also enables lenders to make more informed lending decisions.
- Razorpay: Known primarily as a payment gateway, Razorpay has expanded its services to include lending solutions powered by AI. The company evaluates the creditworthiness of small businesses by analyzing their transaction data and financial health. This allows Razorpay to offer tailored loan products that meet the specific needs of businesses, thereby improving risk management and nurturing business growth.
- KredX: KredX is a platform specializing in invoice discounting and supply chain financing. The company uses AI to assess the credit risk of businesses seeking financing against their invoices. By analyzing various data points, including payment history and business performance metrics, KredX can provide a more accurate risk assessment, leading to better lending decisions and improved financial outcomes for both lenders and borrowers.
- Paytm: Paytm, a leading digital payment platform in India, has integrated AI into its lending services to enhance credit scoring processes. The company analyzes user behavior, transaction history, and other relevant data to evaluate creditworthiness. This enables Paytm to offer personalized loan products and improve the efficiency of its credit assessment processes, ultimately benefiting both the company and its customers.
The Benefits of AI-Driven Credit Scoring
The adoption of AI in credit scoring offers several significant benefits for financial institutions, lenders, and borrowers alike. Some of the key advantages include:
- Improved Accuracy in Credit Risk Assessment: AI-driven credit scoring models can analyze vast amounts of data from multiple sources, providing a more accurate and comprehensive assessment of creditworthiness. This reduces the likelihood of default and enhances the overall quality of lending decisions.
- Enhanced Financial Inclusion: Traditional credit scoring methods often exclude individuals and businesses with limited credit histories or non-traditional financial profiles. AI-driven models, on the other hand, can consider a wider range of data points, making it possible for lenders to extend credit to a broader pool of borrowers. This promotes financial inclusion and helps underserved populations access financial services.
- Personalized Loan Products: AI enables lenders to offer personalized loan products tailored to the specific needs and financial circumstances of borrowers. This improves customer satisfaction and loyalty, as borrowers are more likely to receive loan offers that align with their financial goals and capabilities.
- Reduced Operational Costs: AI-driven credit scoring models can automate many aspects of the credit assessment process, reducing the need for manual intervention and lowering operational costs. This allows financial institutions to streamline their lending processes and allocate resources more efficiently.
- Faster Decision-Making: AI-powered credit scoring models can process and analyze data in real-time, enabling lenders to make faster and more informed lending decisions. This not only enhances the customer experience but also allows lenders to respond more quickly to changing market conditions.
Challenges and Considerations
While the benefits of AI-driven credit scoring are significant, there are also several challenges and considerations that financial institutions and fintech companies must address:
- Data Privacy and Security: The use of AI in credit scoring requires access to vast amounts of personal and financial data, raising concerns about data privacy and security. Financial institutions must ensure that they have robust data protection measures in place to safeguard sensitive information and comply with relevant regulations.
- Bias and Fairness: AI models are only as good as the data they are trained on. If the training data is biased or unrepresentative, the resulting credit scores may also be biased, leading to unfair lending decisions. It is essential for financial institutions to carefully monitor and audit their AI models to ensure that they are fair and unbiased.
- Regulatory Compliance: The use of AI in credit scoring is subject to various regulations and guidelines, depending on the jurisdiction. Financial institutions must ensure that their AI-driven credit scoring models comply with all applicable laws and regulations, including those related to consumer protection and anti-discrimination.
- Transparency and Explainability: One of the challenges of AI-driven credit scoring is the “black box” nature of some AI models, which can make it difficult to explain how a particular credit score was determined. Financial institutions must strive to make their AI models more transparent and provide clear explanations to borrowers about how their credit scores were calculated.
- Integration with Existing Systems: Implementing AI-driven credit scoring models often requires integration with existing systems and processes. Financial institutions must ensure that their AI models are compatible with their current infrastructure and that they can be seamlessly integrated into their operations.
The Future of AI in Credit Scoring
The future of AI in credit scoring looks promising, with continued advancements in machine learning algorithms, big data analytics, and AI-driven decision-making. As financial institutions and fintech companies continue to invest in AI technologies, we can expect to see further improvements in the accuracy, efficiency, and fairness of credit scoring models.
One of the key trends to watch in the coming years is the increasing use of alternative data sources in credit scoring. As AI models become more sophisticated, they will be able to analyze a wider range of data points, including social media activity, online behavior, and even biometric data. This will enable lenders to gain a deeper understanding of borrowers’ creditworthiness and make more informed lending decisions.
Another important trend is the rise of explainable AI (XAI) in credit scoring. As regulators and consumers demand greater transparency in AI-driven decision-making, financial institutions will need to develop AI models that can provide clear and understandable explanations of how credit scores are determined. This will help to build trust in AI-driven credit scoring models and ensure that they are used fairly and responsibly.
Conclusion
AI-driven credit scoring represents a significant advancement in financial risk management, offering numerous benefits for lenders and borrowers alike. By leveraging machine learning algorithms and big data analytics, AI-powered credit scoring models can provide more accurate, efficient, and fair assessments of creditworthiness, ultimately leading to better lending decisions and improved financial outcomes.
However, the adoption of AI in credit scoring also presents several challenges, including issues related to data privacy, bias, and regulatory compliance. Financial institutions and fintech companies must carefully navigate these challenges to fully realize the potential of AI-driven credit scoring.
As the global market for credit scoring services continues to grow, AI-driven solutions are composed to play an increasingly important role in shaping the future of the financial industry. Using these technologies and addressing the associated challenges, financial institutions can experience new opportunities for innovation, improve financial inclusion, and better serve their customers in constantly changing fintech domain.