Transforming Data into Actionable Insights: A Roadmap for AI & Analytics Journey
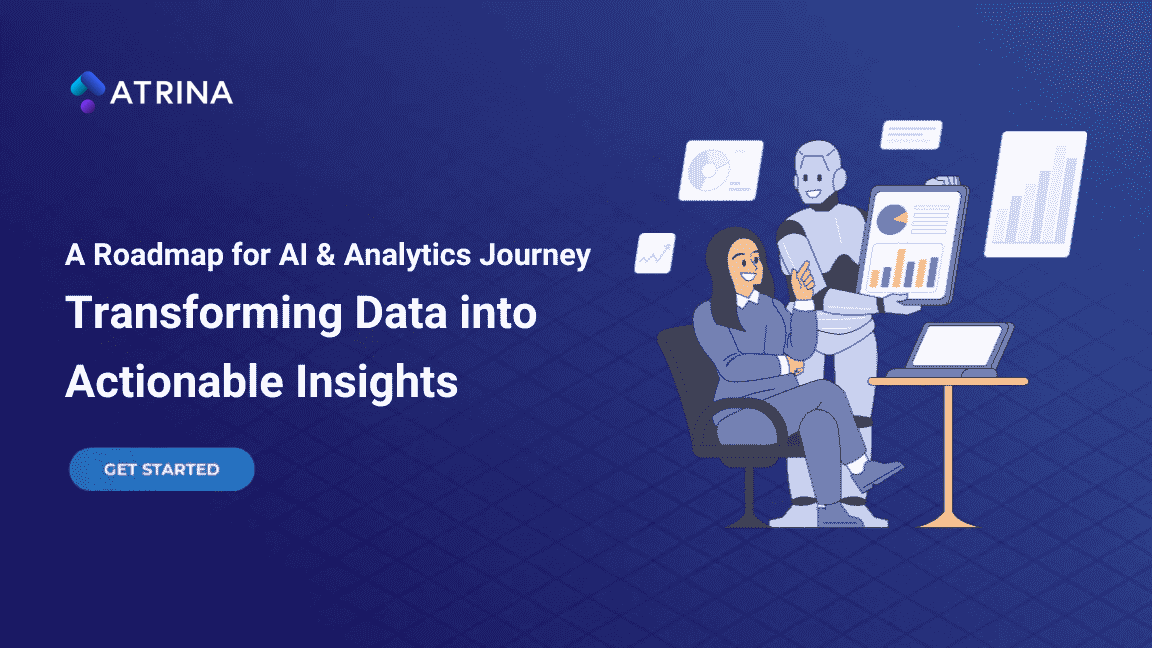
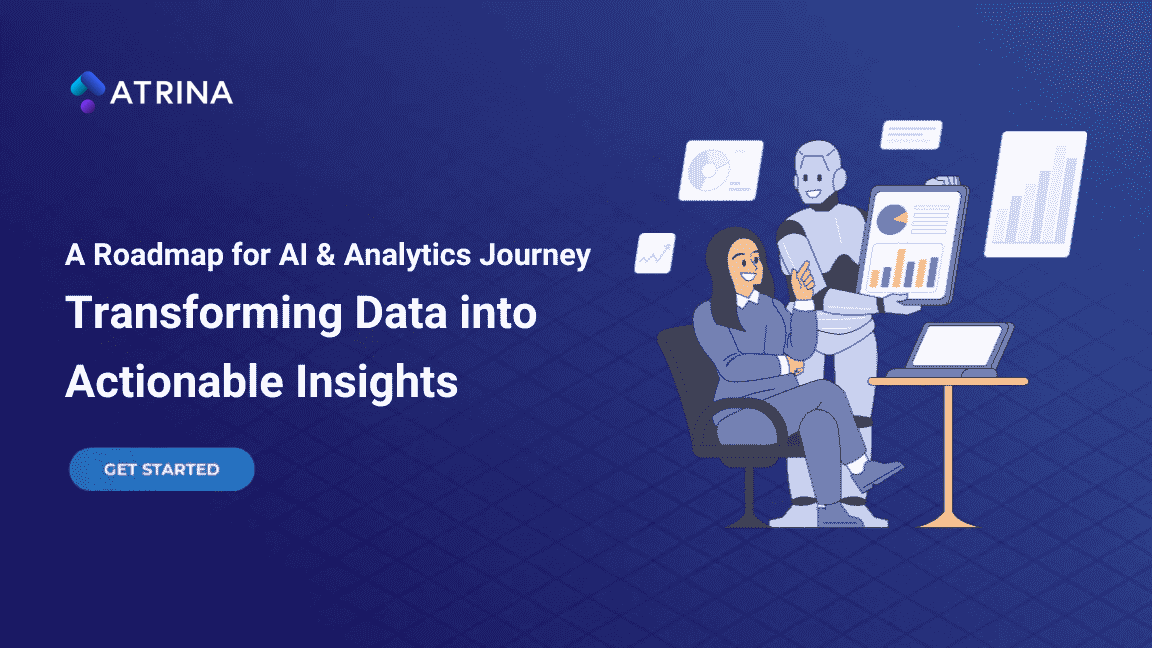
Did you know that by 2025, the global data sphere is projected to reach an astounding 180 zettabytes?
That’s 180 trillion gigabytes of information – an amount so vast it’s difficult to even comprehend. But within this sea of data lies an abundance of insights waiting to be unearthed.
Businesses that successfully integrate analytics and artificial intelligence (AI) stand to gain a sizable competitive advantage.
This comprehensive guide will equip you for the AI & Analytics Journey, exploring the crucial stages involved in transforming raw data into actionable insights that fuel intelligent decision-making and drive business growth.
Defining The AI & Analytics Journey
The AI & Analytics Journey is a strategic roadmap for organizations to leverage data effectively. It’s a cyclical process encompassing data collection, preparation, analysis, model building, deployment, and ultimately, the generation of actionable insights to guide business decisions. This continuous cycle empowers organizations to make smarter choices, optimize operations, improve customer experiences, and gain a competitive edge.
Stages of the AI & Analytics Journey
- Understanding Data Collection
The foundation of the AI & Analytics Journey lies in data collection. Identifying and gathering relevant data from various sources is critical for generating valuable insights. Here are some key aspects to consider:
- Data Sources: Internal databases (CRM, ERP, transaction data), external APIs (social media data, weather data), Internet of Things (IoT) devices (sensor data from equipment).
- Data Quality: Accurate, complete, and consistent data is essential for reliable AI models and insights. Techniques like data cleaning and validation ensure data quality.
- Data Security and Compliance: Implement robust security measures to protect sensitive data and comply with relevant data privacy regulations like GDPR and CCPA.
- Data Preparation and Cleaning
Raw data is rarely perfect. Before analysis, data preparation involves cleaning, normalization, and transformation to ensure consistency and usability. This stage may involve:
- Data Cleaning: Identifying and rectifying errors, inconsistencies, and missing values.
- Data Normalization: Ensuring data formats are consistent across different data sets.
- Data Transformation: Transforming data into a format suitable for analysis (e.g., scaling numerical data).
- ETL Processes: Extract, Transform, Load (ETL) processes automate data preparation tasks for efficient data management.
- Data Wrangling: An iterative process of data exploration and manipulation to prepare data for analysis.
- Model Building and Training
Once your data is clean and prepared, it’s time to build AI models. These models are algorithms trained on historical data to identify patterns and make predictions. Here’s what you need to know:
- Machine Learning Models: There are various types of machine learning models suited for different tasks (e.g., classification, regression, clustering). Understanding your use case is crucial for selecting the right model.
- Model Training: The AI model learns from historical data to identify patterns and relationships. Training data quality significantly impacts model performance.
- Model Validation: Evaluating the model’s performance on unseen data ensures it generalizes well and doesn’t overfit the training data.
- Hyperparameter Tuning: Fine-tuning model parameters can significantly improve its accuracy. Consider using techniques like grid search or randomized search.
- Model Selection: Compare the performance of different models to choose the one best suited for your specific needs.
- AI Model Deployment and Integration
Once you have a well-trained model, it’s time to deploy it into production. This involves:
- Model Deployment Strategies: Consider cloud-based deployment for scalability and ease of access. Alternatively, on-premise deployment may be necessary for sensitive data.
- Scalability: Ensure your infrastructure can handle increasing data volumes and user requests as your AI model scales.
- Monitoring: Continuously monitor model performance to detect any deterioration in accuracy and identify opportunities for improvement.
- Integration with Existing Systems: Seamlessly integrate your AI model with existing business systems for efficient data flow and insights utilization.
- Generating Actionable Insights
The ultimate goal of the AI & Analytics Journey is to generate actionable insights from data. This involves:
- Data Visualization: Present data in an easily digestible format using dashboards, charts, and graphs to facilitate communication and interpretation of insights.
- Storytelling with Data: Go beyond just presenting data; tell a story with it, highlighting key findings and their implications for decision-making.
This stage translates complex data into actionable knowledge that permits leaders to make informed decisions based on evidence, not guesswork. For example, using AI to predict customer churn risk and implement targeted retention campaigns or leveraging insights to personalize product recommendations for a more satisfying shopping experience.
Entry Points for Different Audiences
The AI & Analytics Journey caters to individuals with varying levels of expertise:
- Beginners: Start with foundational concepts like data types, basic data analysis techniques, and data visualization tools. Numerous online resources, tutorials, and introductory courses can equip you with the essential knowledge to embark on your data analytics journey.
- Intermediate Users: Deepen your understanding by exploring data cleaning techniques, machine learning algorithms, and model training methodologies. Consider enrolling in online courses or attending workshops on specific AI and analytics tools like Python or R.
- Advanced Practitioners: Delve into advanced topics like deep learning architectures, natural language processing, and reinforcement learning. Engage in hackathons and Kaggle competitions to test your skills and stay at the forefront of the AI landscape.
Best Practices and Tips for Your AI & Analytics Journey
Optimizing your AI & Analytics Journey requires a strategic approach and continuous improvement. Here are some valuable best practices and tips:
- Data Governance: Implement a data governance framework to ensure data quality, access control, and responsible data utilization. This builds trust and transparency within your organization.
- Model Explainability: Ensure your AI models are interpretable and understandable. Techniques like LIME (Local Interpretable Model-Agnostic Explanations) help explain model predictions, nurturing trust and regulatory compliance.
- Ethical Considerations: Be mindful of ethical considerations throughout the AI & Analytics Journey. Address potential biases in data sets and algorithms, and ensure responsible AI development and deployment.
- Continuous Improvement: The AI & Analytics Journey is an ongoing process. Regularly evaluate your data infrastructure, models, and analytical tools. Adopt a culture of experimentation and innovation to adapt to evolving technologies and business needs.
- Invest in Your People: Upskilling your workforce is crucial. Provide training programs on data literacy, data analysis techniques, and relevant AI tools. Building a data-driven culture empowers employees at all levels to leverage data for informed decision-making.
The Disruptive Potential of the AI & Analytics Journey
The AI & Analytics Journey signifies a shift from intuition-based decision-making to data-driven strategies. With AI and analytics, organizations can realize a wealth of insights, optimize operations, gain a competitive edge, and achieve sustainable growth.
Are you prepared to use data to reinvent the way you operate? Our all-inclusive AI & Analytics solutions can assist you at every step of the process, from gathering and cleaning data to creating effective models and producing insights that can be put to use.
Here’s what we offer:
- Expert guidance: Our team of data scientists and engineers will partner with you to design a customized AI & Analytics strategy aligned with your specific business goals.
- Seamless implementation: We leverage cutting-edge technologies to ensure efficient data management, model development, and integration with your existing systems.
- Actionable results: We don’t just deliver insights – we help you translate them into real-world actions that drive measurable business value.
Don’t wait to get started!
Contact us today for a free consultation and reveal the hidden potential within your data.