Building a Culture of Data-Driven Decisions with AI & Analytics
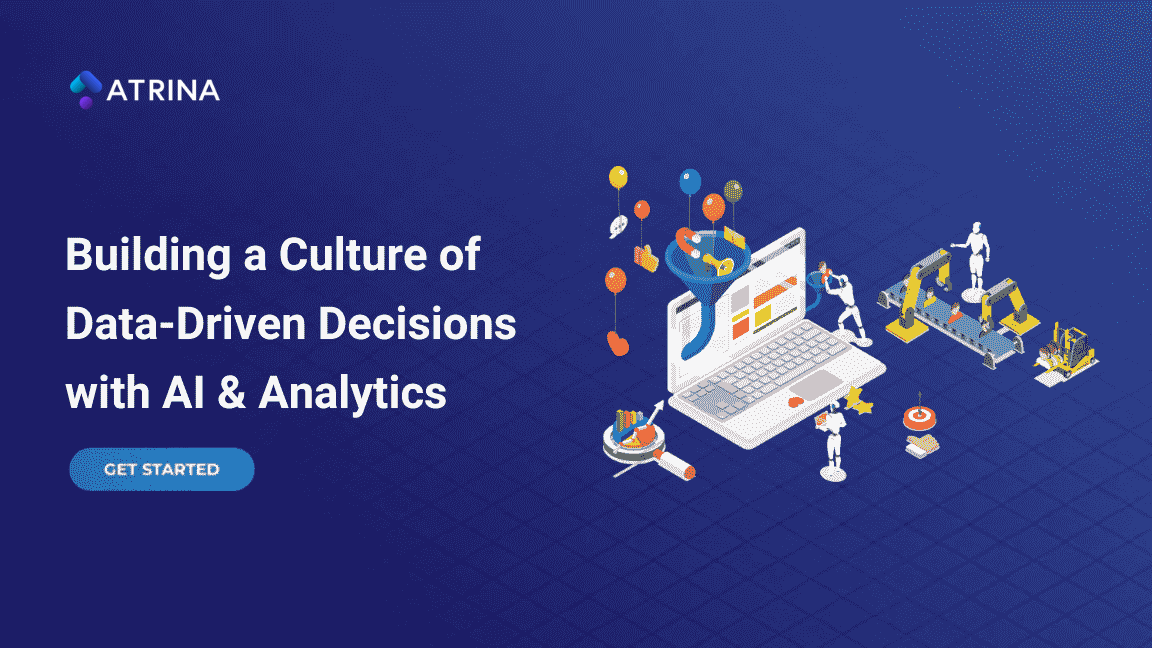
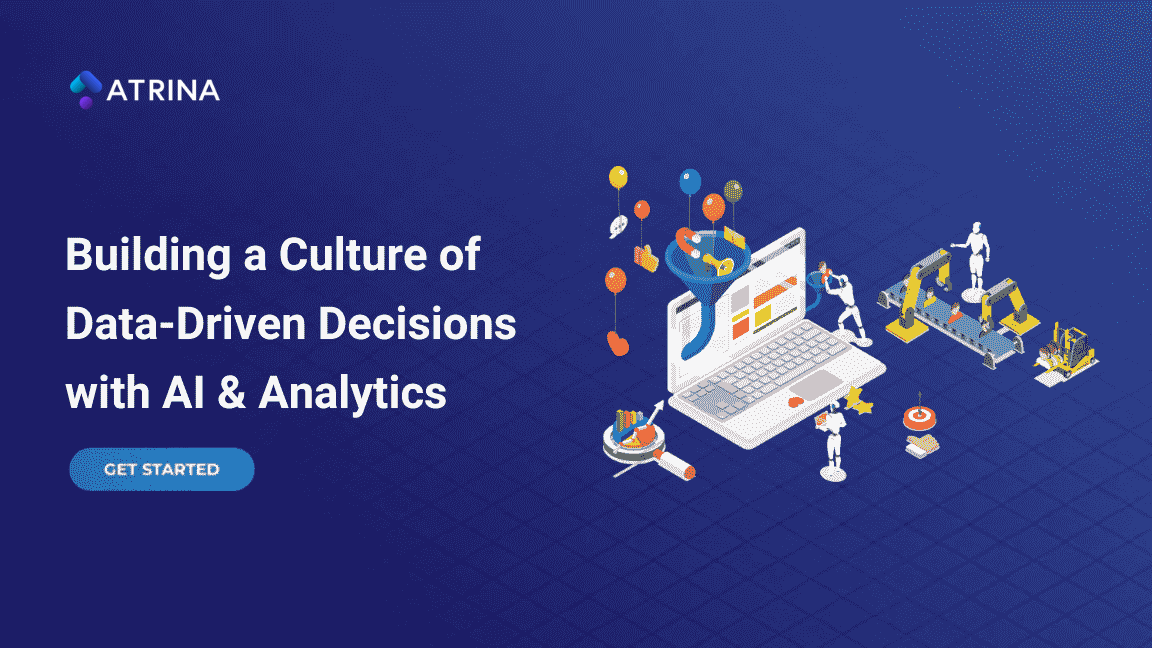
Data is no longer just information – it’s the lifeblood of intelligent decision-making.
Organizations that leverage data effectively gain a significant competitive edge. This is where building a culture of data-driven decision making (DDDM) comes into play.
This comprehensive guide dives deep into the power of DDDM, exploring how AI and analytics empower organizations to make informed choices, achieve sustainable growth, and stay ahead of the curve.
Data-Driven Decisions: The Key to Success
Data-driven decision making (DDDM) is a strategic approach that utilizes data analysis and insights to guide business decisions. It involves collecting, storing, analyzing, and interpreting data to understand customer behavior, market trends, operational efficiency, and more. This data-centric approach empowers businesses to move beyond intuition and guesswork, leading to:
- Improved Decision Quality: Data provides factual evidence to support decisions, reducing bias and nurturing objectivity. Studies have shown that data-driven companies are 23 times more likely to outperform their competitors in terms of profitability.
- Enhanced Efficiency and Productivity: Data-driven insights help organizations streamline processes, identify bottlenecks, and optimize resource allocation. For instance, a data-driven approach to logistics can significantly reduce delivery times and optimize delivery routes.
- Increased Customer Satisfaction: Data analysis helps businesses understand customer needs and preferences, enabling them to personalize products, services, and marketing strategies. This leads to higher customer satisfaction and loyalty, which translates to increased revenue and market share.
- Reduced Risks and Costs: Data helps predict potential issues and identify areas for improvement, allowing businesses to proactively address challenges and minimize costs. Predictive maintenance in manufacturing, for example, can prevent costly equipment failures and downtime.
- Data-Backed Innovation: Data can spark new ideas and opportunities, accelerating innovation and propelling businesses forward. Companies can leverage data to identify market gaps, develop new products and services, and improve existing offerings.
The Role of AI and Analytics in Data-Driven Decisions
The power of DDDM is further amplified by the capabilities of Artificial Intelligence (AI) and analytics. Here’s how these technologies work together:
- AI and Machine Learning (ML): These technologies enable businesses to analyze massive datasets, identify complex patterns, and make predictions that would be impossible with traditional methods. Machine learning algorithms can analyze customer behavior data to predict churn risk or identify potential high-value customers.
- Advanced Analytics: Techniques like statistical analysis, predictive modeling, and forecasting provide deeper insights from data, uncovering hidden trends and connections. Businesses can use advanced analytics to identify factors influencing customer purchasing decisions or predict market fluctuations.
- Data Visualization: Dashboards and interactive visualizations transform complex data sets into easily digestible formats, facilitating communication and decision-making across all levels of an organization. Compelling visualizations can help stakeholders quickly grasp key insights and make informed decisions.
Building a Data-Driven Culture: A Roadmap to Success
Establishing a DDDM culture requires a strategic approach and organizational commitment. Here are the key steps to get you started:
- Leadership Buy-in: Executive leaders need to champion the data-driven approach and set a clear vision for data utilization. Leaders must demonstrate their commitment to DDDM by allocating resources, investing in data infrastructure, and encouraging data-driven decision making throughout the organization.
- Define Data Strategy: Align data collection, analysis, and utilization with your business goals and objectives. Develop a data strategy that outlines what data you need to collect, how you will analyze it, and how the insights will be used to achieve your business objectives.
- Invest in Data Infrastructure: Build a robust infrastructure for data storage, management, and security. This includes selecting appropriate data storage solutions, implementing data governance policies, and ensuring data security and compliance with relevant regulations.
- Develop Data Literacy: Train employees at all levels to understand data concepts and use data insights effectively. This includes providing training on data interpretation, data visualization, and basic data analysis skills. Building a data-literate workforce is crucial for ensuring everyone can leverage data for decision-making.
- Promote Collaboration: Nurture a culture of open communication and information sharing around data-driven insights. Break down silos between departments and encourage collaboration to ensure everyone has access to the data they need to make informed decisions.
- Empower Data Champions: Identify and empower data champions who can advocate for DDDM across different departments. Data champions can act as internal evangelists for data-driven decision making and provide support to colleagues who are less familiar with data analysis.
Implementing AI & Analytics Solutions
The modern business landscape offers a wide range of AI and analytics tools and platforms tailored to diverse needs.
Here are some key considerations when implementing AI and analytics solutions:
- Data Integration: Seamlessly connect data sources from various systems like CRM, ERP, and marketing automation platforms. This allows you to create a unified view of your data and gain holistic insights. There are various data integration tools and techniques available to help you achieve this.
- Data Cleaning and Preparation: Ensure data quality by identifying and addressing errors or inconsistencies that can skew results. Data cleaning tasks may involve correcting missing values, removing duplicates, and standardizing data formats. Investing in data quality processes is essential for ensuring the reliability and validity of your data analysis.
- Data Analysis and Modeling: Choose the right AI and analytics tools based on your specific goals. Tools like:
- Sentiment analysis: Analyzes customer reviews, social media posts, and other text data to understand customer sentiment and opinions.
- Risk prediction: Identifies potential risks such as financial fraud or customer churn, allowing businesses to take preventative measures.
- Customer segmentation: Groups customers based on shared characteristics, enabling targeted marketing campaigns and personalized experiences.
- Prescriptive analytics: Recommends specific actions to take based on data analysis, helping businesses optimize operations and marketing efforts.
- Data Visualization and Storytelling: Effectively communicate insights through compelling visuals and clear narratives. Utilize data visualization tools to create dashboards, charts, and graphs that make it easy for stakeholders to understand complex data. Don’t just present data; tell a story with it, highlighting key findings and their implications for decision-making.
Overcoming Challenges on the Road to Data-Driven Decisions
While the benefits of DDDM are substantial, there are challenges companies need to address:
- Data Quality: Inconsistent or inaccurate data can lead to misleading insights. Robust data governance policies are essential to ensure data quality and integrity. This includes establishing data ownership, defining data standards, and implementing data quality checks.
- Privacy Concerns: Data security and privacy compliance are paramount. Organizations must ensure transparency and build trust with users. Develop a data privacy policy that outlines how you collect, store, and use user data. Furthermore, implement appropriate security measures to protect user data from unauthorized access.
- Cultural Resistance: People may be resistant to change. Communication, training, and showcasing the value of DDDM are crucial. Effectively communicate the benefits of data-driven decision making to address employee concerns and encourage buy-in. Provide training to help employees develop data skills and confidence in working with data.
- Skills Gap: Organizations may lack the necessary data science and analytics skills to leverage AI and analytics effectively. Invest in training and development programs to upskill your workforce or consider partnering with data science experts for specialized tasks.
Metrics and Measurement: Tracking Your Progress
To ensure your DDDM efforts are successful, it’s crucial to track your progress. Here are some key metrics to consider:
- Data Quality Metrics: Measure the accuracy, completeness, and consistency of your data.
- Data Adoption Metrics: Track how many users are accessing and using data insights for decision-making.
- Business Outcome Metrics: Align your data analytics initiatives with specific business goals and track how data-driven decisions impact those goals. For example, if you’re using data to personalize marketing campaigns, track metrics like conversion rates and customer acquisition costs.
Building a Sustainable Data Strategy for Growth
Building a DDDM culture demands a long-term vision. Here’s how to create a sustainable data strategy:
- Align Data Strategy with Business Objectives: Data initiatives should directly support your overarching business goals. Don’t implement data analytics for the sake of it; ensure it has a clear purpose and contributes to your overall business strategy.
- Adopt a Continuous Improvement Mindset: Regularly evaluate data governance, infrastructure, AI models, and analytical tools, and adapt to evolving needs. The data landscape is constantly changing, so it’s important to be flexible and adaptable in your approach.
- Invest in Data Governance: Develop a framework for data ownership, access control, and security to ensure responsible data utilization. Data governance policies are essential for protecting data privacy, ensuring compliance with regulations, and nurturing trust with stakeholders.
- Develop a Data-Driven Mindset: Encourage a culture of experimentation and data-driven learning across all levels of the organization. Empower employees to ask questions, explore data, and identify opportunities for data-driven decision making.
- Invest in Ongoing Training and Skill Development: Equip your workforce with the skills and knowledge to leverage data effectively. Provide ongoing training programs to help employees develop data literacy, data analysis skills, and expertise in using data visualization tools.
Becoming a Data-Driven Leader
Building a culture of data-driven decision making (DDDM) is a transformative journey. Adopting AI and analytics empowers organizations to realize the true potential of their data, nurturing a competitive edge and driving sustainable growth. By actively implementing the strategies and considerations outlined in this comprehensive guide, you can pave the way for a data-driven future and solidify your position as a leader in the ever-evolving business world.
FAQs: Frequently Asked Questions About Data-Driven Decision Making (DDDM)
Here are ten frequently asked questions to shed light on DDDM and its implementation:
- What are the different types of data used in DDDM?
There are various types of data used in DDDM, including structured data (numerical data stored in databases), unstructured data (textual data like emails and social media posts), and semi-structured data (data with some organization but not a rigid format like XML). Organizations leverage a combination of data types to gain a holistic view of their operations and customers.
- Is DDDM just for big businesses?
No, DDDM is beneficial for businesses of all sizes. Even small businesses can leverage data from sales figures, customer interactions, and website analytics to make more informed decisions. There are also affordable AI and analytics tools available to cater to the needs of smaller companies.
- How long does it take to implement DDDM?
The timeframe for implementing DDDM varies depending on the size and complexity of your organization. However, it’s an ongoing process rather than a one-time initiative. Building a data-driven culture requires continuous learning, adaptation, and refinement of your approach.
- What are the biggest challenges to implementing DDDM?
Common challenges include ensuring data quality, addressing privacy concerns, overcoming cultural resistance to change, and developing the necessary data skills within the workforce. Proactive strategies and ongoing communication are key to mitigating these challenges.
- How can I measure the success of DDDM initiatives?
Track key metrics aligned with your business goals. This could include data quality metrics, data adoption metrics, and business outcome metrics like increased sales, improved customer satisfaction, or reduced costs.
- What are some examples of how AI is used in DDDM?
AI is used for various tasks in DDDM, such as analyzing customer sentiment from social media data, predicting customer churn risk, or identifying fraudulent transactions. Machine learning algorithms can uncover hidden patterns and trends in data, leading to valuable insights.
- What are the benefits of data visualization in DDDM?
Data visualization transforms complex data sets into charts, graphs, and dashboards, making it easier for everyone in the organization to understand data and communicate insights effectively. Visualizations can significantly improve data accessibility and decision-making across all levels.
- How can I ensure data privacy when using DDDM?
Transparency is crucial. Develop a data privacy policy that outlines how you collect, store, and use user data. Furthermore, implement appropriate security measures to protect user data and comply with relevant data privacy regulations.
- What skills are needed for a data-driven workforce?
Data literacy, basic data analysis skills, and the ability to interpret data visualizations are essential. Additionally, depending on the specific role, skills in data mining, statistics, or programming may be beneficial.
- Where can I learn more about DDDM and data analytics?
Numerous online resources are available, including industry publications, online courses, and webinars offered by data analytics companies. Consider attending industry conferences and workshops to stay updated on the latest trends and best practices in DDDM.
Good Read: How Can Products Study Their Users Better by Using New Tech? (AI/ML)
Ready for your data-driven journey? We can help!
Contact our team of AI and analytics experts to discuss how we can develop a solution to meet your specific business needs. Whether you’re just starting out or looking to take your DDDM efforts to the next level, we have the expertise and resources to help you achieve your goals.